Geospatial Analysis
Several tools are provided in SADA for performing a geospatial analysis.
These tools include methods for measuring spatial correlation among data,
modeling spatial correlation, and producing concentration, risk, probability,
variance, and cleanup maps. Among these tools includue seven options:
- ordinary kriging,
- indicator kriging,
- cokriging
- inverse distance,
- natural neighbor,
- nearest neighbor.
- import your own
and two simulation methods.
The last option "import your own" may be of particular interest. SADA has a couple of industry
standard formats (ASCII Grid, FLOAT Grid) as well as a SADA format (fairly low requirements for importing 3d models)
for importing your own models. When you import your own models, they can be used in line with the other modeling
and decision analysis features just as if they were generated in SADA.
Basic estimation methods such as inverse distance, natural neighbor, and nearest neighbor produce a single estimation map
for a given set of model parameters. Natural neighbor is particularly easy for novice users as it requires no
input from the user (based on simple geometries). Kriging goes beyond estimation to provide a model of uncertainty that is useful in determining
how well understood estimation values are. Often kriging results are very smoothed. This can be aleviated by
the use of heterogenous secondary data (see below) to better inform the kriging model about local variations. In
addition, simulation models provide full access to point and joint uncertainty assessments
and also produce more heterogeous maps the attribute (contaminant) of interest.
The following shows some typical applications of SADA geospatial models. In the first image we see an evaluation of
correlation structure by creating a variogram map (rose) in search of anisotropic conditions and then the fitting of a model
through experimental variogram results. The user has some assistance in this often challenging aspect of a
geostatistical analysis with recommendations, autofitting, etc.
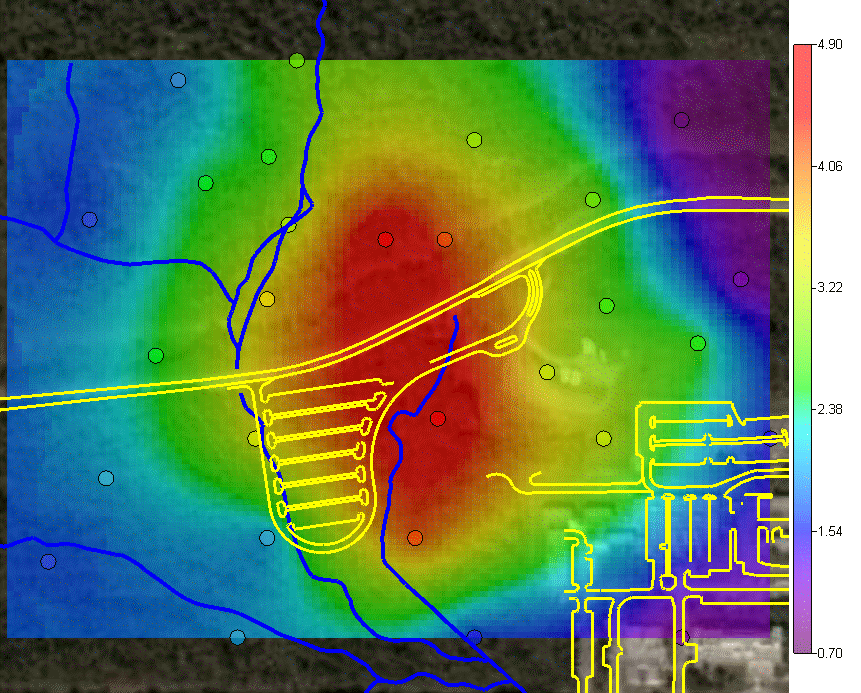
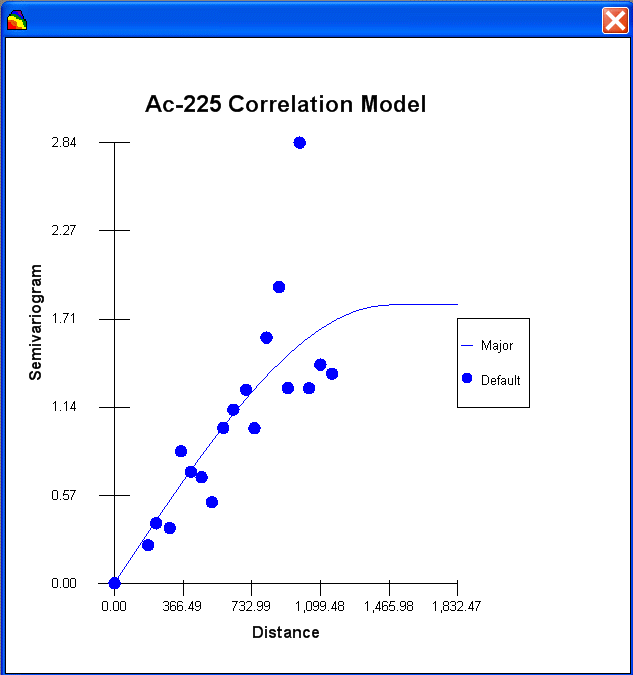
One product of a geospatial analysis is the contour map.
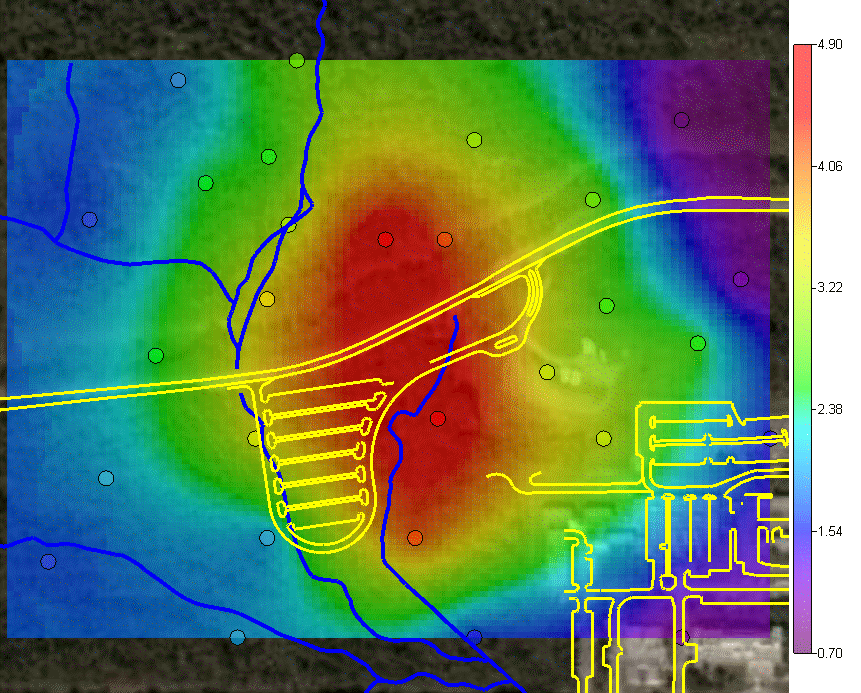
The local estimates of uncertainty in kriging (and simulation) translate into uncertainties about the final decision.
In the following area of concern map, the exact boundary of the problem area is unknown and characterized by the green zone
surrounding the gray (contaminated) zone. The think black line represents the best estimated boundary line given
the current state of information and callibration of the model.
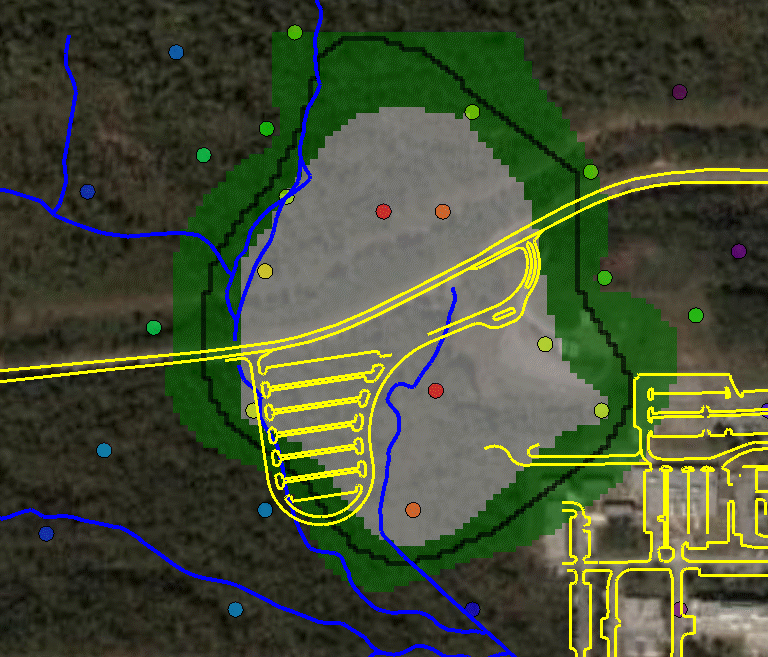
These bands of uncertainty serve as a jumping off point for discussion
and implementation of secondary sampling designs
that seek to optimally locate samples to better inform a particular decision or improve local geographical information.
In many situations, there is insufficient data to reasonably build a geospatial model of any kind. Maps of these types
of geospatial models are very, very smooth.
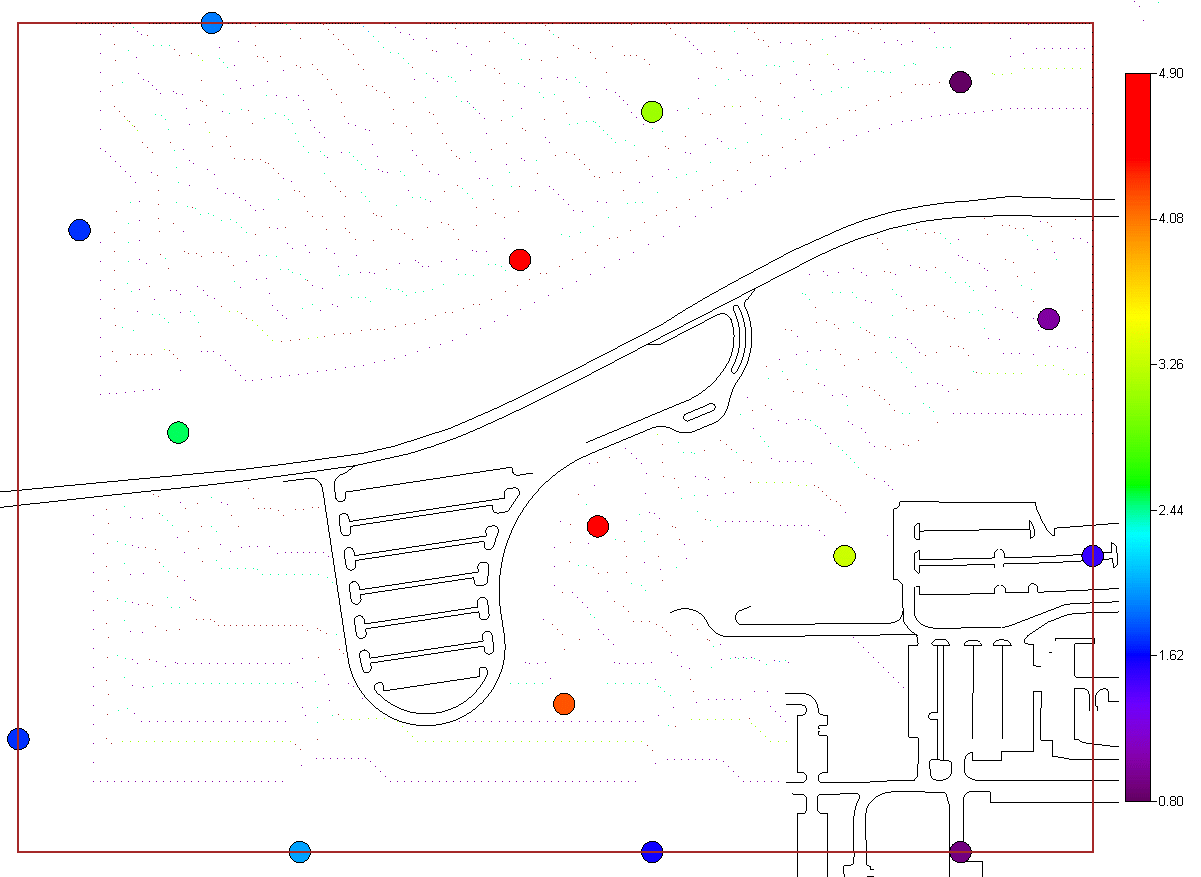
Kriging these results produces the following map.
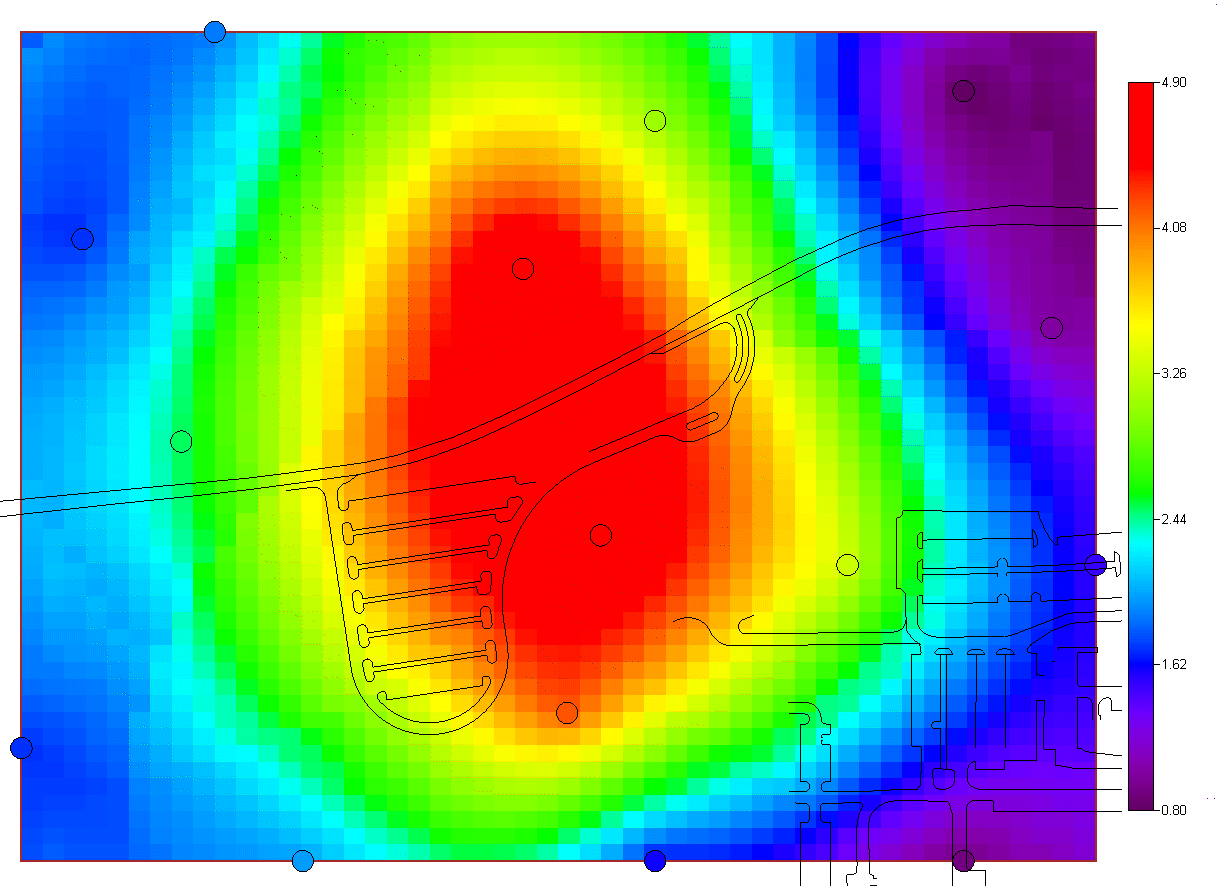
This is not to imply that such maps have no value. In fact, for many environmental characterizations there
may never be more samples than the number found here and the state of information is limited by the availability of
data.
In some cases however, secondary information may be readily available. This secondary information is not per se a measurement
of the attribute or contaminant of interest. However, there may exist a correlation between this
secondary form of information and the primary information at hand. Examples of secondary data types include
radiological walk over surveys, borehole scans, XRF, geological models, traditional geophysical measurements of
the subsurface, and many other types of field detection methods. This information may be less exensive to collect and
therefore more abundant.
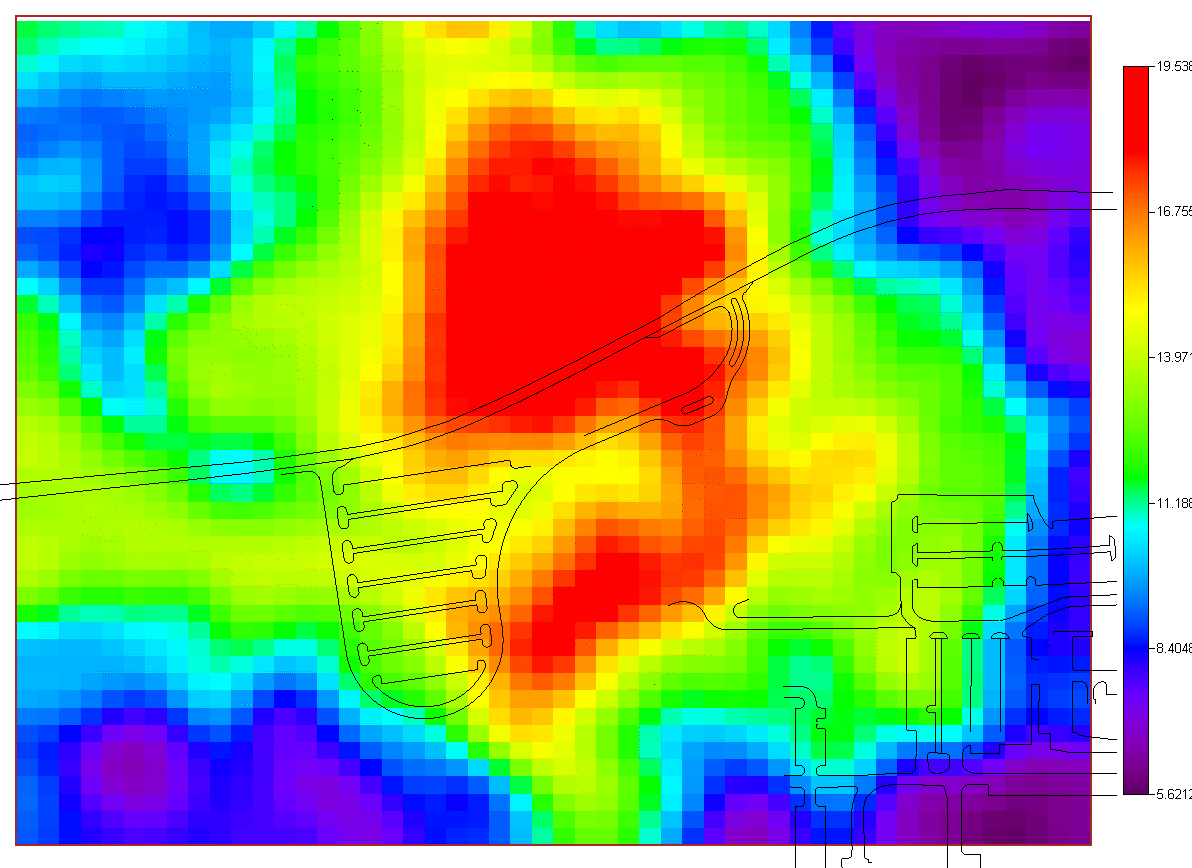
This information can be explicitly used in the model to better inform them model, produce
more heterogeneous maps, and reduce model/decision uncertainty. The following image shows the addition of a
adding a densely collected geophysical survey to the analysis.
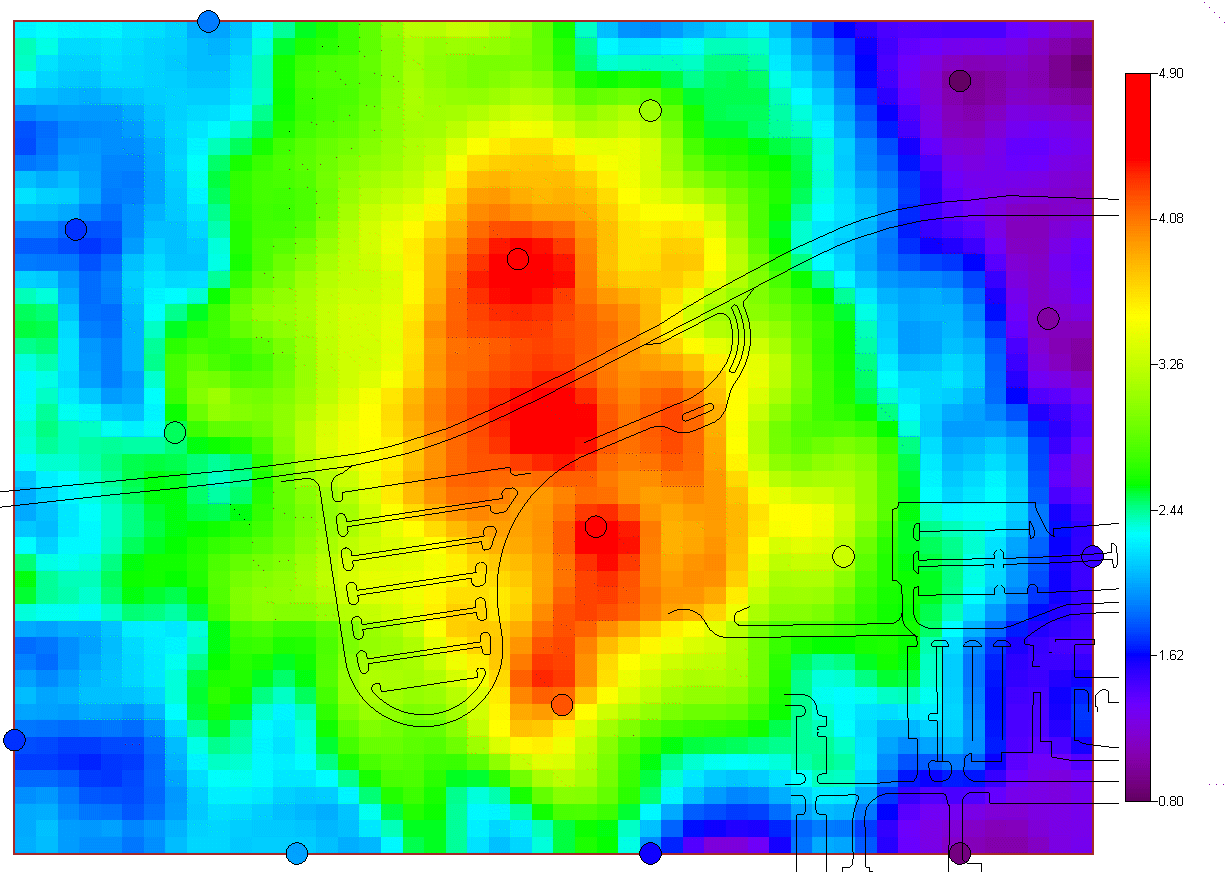
|